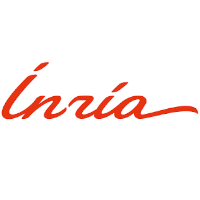
13 Dec PostDoc – Federated Statistical Learning for New Generation Meta-Analyses
JOB OFFER Postdoctoral position
Federated Statistical Learning for New Generation Meta-Analyses of Large-scale and Secured Biomedical Data (Fed-BioMed)
Project Description.
The project Fed-BioMed focuses on methodological and technical advances towards the development of a novel generation of federated learning methods for the analysis of private and large-scale multi-centric biomedical data. The project has a specific focus on the efficient federation of frameworks robust to data heterogeneity and uncertainty, and tackles the following scientific challenges:
- Methodological. Extending the federated paradigm to novel scalable approaches to probabilistic modeling and prediction from siloed data.
- Technical. Developing our federated learning framework through a self-contained system that can be securely deployed across different centers and collaborators (fedbiomed.gitlabpages.inria.fr).
- Translational. Demonstrating federated learning on two applications:
- Discovering novel genetic underpinnings of neurological and psychiatric disorders, and
- Prediction of response to immunotherapy from the analysis of federated lung imaging data.
During the project the candidate will:
- Develop learning methods for federated analysis for private and distributed data;
- Deploy advanced statistical learning methods into a wide range of biomedical/clinical applications;
- Interact with INRIA researchers and engineers, and participate to the scientific life of the team;
Required competences.
Demonstrable experience in some of the following topics (the more the better):
- Statistics, Bayesian Modeling;
- Optimization, Distributed Computing;
- Python and PyTorch/TensorFlow;
- Biomedical Data Analysis;
- Signal Processing;
Strong communication abilities are necessary, as well as motivation in taking responsibilities (e.g. supervision, organization of scientific events).
Hosting Research Group.
Epione team (Inria), located in the tech park of Sophia Antipolis (France). The longstanding research activity of our group revolves around the analysis and treatment of biomedical data, with a focus in machine learning, medical imaging, computational anatomy and computational physiology. Over the past twenty years the group developed innovative approaches in image processing, statistical learning and patient-specific biophysical modeling, with translation to the clinical domain, and to the creation of several biotech startups. The group is currently composed by 6 permanent researchers, several postdoc fellows and research engineers, and by more than 20 PhD students.
Offer.
- 18 months contract
- Salary upon experience
- Starting date: asap
Contact:
Email: marco.lorenzi@inria.fr
References:
- Yann Fraboni, Richard Vidal, Laetitia Kameni, Marco Lorenzi. Clustered Sampling: LowVariance and Improved Representativity for Clients Selection in Federated Learning. Proceedings of the 38th International Conference on Machine Learning (ICML 2021).
- Yann Fraboni, Richard Vidal, and Marco Lorenzi. Free-rider Attacks on Model Aggregation in Federated Learning. In AISTATS 2021.
- Irene Balelli, Santiago Silva, and Marco Lorenzi. A Probabilistic Framework for Modeling the Variability Across Federated Datasets of Heterogeneous Multi-View Observations. International Conference of Information processing in Medical Imaging, IPMI 2021.
- Santiago Silva, Boris Gutman, Barbara Bardoni, Paul M Thompson, Andre Altmann, Marco Lorenzi. Multivariate Learning in Distributed Biomedical Databases: Meta-analysis of Large-scale Brain Imaging Data. IEEE International Symposium on Biomedical Imaging (ISBI), Venice, 2019.
- Marco Lorenzi, Andre Altmann, Boris Gutman, et al. Susceptibility of brain atrophy to TRIB3 in Alzheimer’s disease: Evidence from functional prioritization in imaging genetics. Proceedings of the National Academy of Sciences of the United States of America (PNAS). March 20, 2018. 115 (12) 3162-3167.
Please click here to learn more.
Sorry, the comment form is closed at this time.