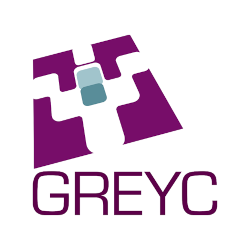
05 Jun PhD Position – Frugal AI for Image Segmentation
PhD Position – University of Caen, CNRS GREYC Laboratory
Frugal AI for image segmentation
Topic
The advent of deep learning has been a real tsunami in the machine learning community, leading to results, especially in computer vision, that we would not have expected a few years before. For many vision tasks, the performances of deep learning algorithms have become equivalent or even superior to human performances.
However, these results have been obtained at the cost of ever-increasing use of resources such as : the size of the model, the time and energy needed to train them, with ever-larger databases and ever-higher annotation requirements. This increase in resource requirements has major drawbacks, related to the impact that ML has on the environment, the difficulty to implement models on embedded architectures, or the challenges raised when models have to be trained on tasks for which little training data is available.
These observations have very recently led some authors [1, 2] to introduce the concept of frugal machine learning and to define what a frugal machine learning methodology should be, and how to evaluate frugality.
In this dissertation, we will study frugality in the context of AI for image segmentation [3]. The objective will be to propose frugal models that can provide efficient results while being structured to provide a reduced time and space complexity. More precisely, we will consider several aspects of frugality and take inspiration from the following recent works : i) the conception of lightweight models by design [4, 5] ii) the compression of existing models [6] iii) the pruning of existing segmentation models [4, 7] iv) frugality on image label and zero shot image segmentation [8, 9].
Qualifications
- Candidates must have an MSc or engineering degree in a field related to computer science, electrical engineering, or applied mathematics, with strong programming skills (in particular with deep learning frameworks).
- Experience with image processing will be a plus.
- Candidates are expected to have abilities to write scientific reports and communicate research results at conferences in English.
Information and application
The position is starting as soon as possible with a salary of 32kEuros gross, and will be located in Caen, France.
Applications should include the following documents in electronic format :
- A short motivation letter stating why you are interested in this project,
- A detailed CV describing your past research background related to the position
- The transcripts for master degrees. iv) The contact information for three references (do not include the reference letters with your applications as we will only ask for the reference letters for short-listed candidates)
Please send your application package to frederic.jurie@unicaen.fr and olivier.lezoray@unicaen.fr.
Ideally located in the heart of Normandy, two hours from Paris and just 10 minutes away from the beaches, Caen, William the Conqueror’s hometown, is a lively and dynamic city.
Références
- Lingjiao Chen, Matei Zaharia, and James Y. Zou, “FrugalML : How to use ML Prediction APIs more accurately and cheaply,” in Advances in Neural Information Processing Systems 33 : Annual Conference on Neural Information Processing Systems 2020, NeurIPS 2020, December 6-12, 2020, Virtual, Hugo Larochelle, Marc’Aurelio Ranzato, Raia Hadsell, Maria-Florina Balcan, and Hsuan-Tien Lin, Eds., 2020.
- Mikhail Evchenko, Joaquin Vanschoren, Holger H. Hoos, Marc Schoenauer, and Michèle Sebag, “Frugal Ma- chine Learning,” arXiv :2111.03731 [cs, eess], Nov. 2021.
- Shervin Minaee, Yuri Y. Boykov, Fatih Porikli, Antonio J Plaza, Nasser Kehtarnavaz, and Demetri Terzopoulos, “Image segmentation using deep learning : A survey,” IEEE Transactions on Pattern Analysis and Machine Intelligence, pp. 1–1, 2021.
- Linjie Wang, Quan Zhou, Chenfeng Jiang, Xiaofu Wu, and Longin Jan Latecki, “DRBANET : A Lightweight Dual- Resolution Network for Semantic Segmentation with Boundary Auxiliary,” arXiv :2111.00509 [cs], Oct. 2021.
- Enze Xie, Wenhai Wang, Zhiding Yu, Anima Anandkumar, Jose M. Alvarez, and Ping Luo, “Segformer : Simple and efficient design for semantic segmentation with transformers,” CoRR, vol. abs/2105.15203, 2021.
- Moonjung Eo, Suhyun Kang, and Wonjong Rhee, “A Highly Effective Low-Rank Compression of Deep Neural Networks with Modified Beam-Search and Modified Stable Rank,” arXiv :2111.15179 [cs], Nov. 2021.
- Wei He, Meiqing Wu, Mingfu Liang, and Siew-Kei Lam, “Cap : Context-aware pruning for semantic segmentation,” in 2021 IEEE Winter Conference on Applications of Computer Vision (WACV), 2021, pp. 959–968.
- Mengde Xu, Zheng Zhang, Fangyun Wei, Yutong Lin, Yue Cao, Han Hu, and Xiang Bai, “A Simple Baseline for Zero-shot Semantic Segmentation with Pre-trained Vision-language Model,” arXiv :2112.14757 [cs], Dec. 2021.
- Maxime Bucher, Tuan-Hung Vu, Matthieu Cord, and Patrick Pérez, “Zero-shot semantic segmentation,” in Advances in Neural Information Processing Systems 32 : Annual Conference on Neural Information Processing Systems 2019, NeurIPS 2019, December 8-14, 2019, Vancouver, BC, Canada, Hanna M. Wallach, Hugo Larochelle, Alina Beygelzimer, Florence d’Alché-Buc, Emily B. Fox, and Roman Garnett, Eds., 2019, pp. 466–477.
Please click here to learn more.
Sorry, the comment form is closed at this time.